Artificial Intelligence is moving at breakneck speed, and Retrieval-Augmented Generation (RAG) is no exception! Gone are the days when RAG simply meant fetching a document and generating a response. In 2025, RAG has transformed into a sophisticated, adaptive AI framework that integrates structured and unstructured data, delivering highly refined, context-aware results.
Let’s dive deep into how RAG is evolving and explore the groundbreaking innovations shaping the next generation of knowledge retrieval and generation.
The Key Variants of RAG in 2025
As AI becomes more specialized, RAG architectures have diversified to handle different data sources, query complexities, and real-time applications. Here are the six major RAG types leading the way:
1. Standard (Naive) RAG – The Traditional Model
This is the most basic form of RAG, where the system retrieves documents from a predefined dataset and generates responses based on the retrieved content. While functional, it lacks deeper reasoning and advanced filtering techniques.
2. Retrieve-and-Rerank RAG – Precision Enhanced
By implementing advanced re-ranking mechanisms, this model significantly improves the quality of retrieved information, ensuring that only the most relevant documents contribute to response generation.
3. Multimodal RAG – Beyond Text Retrieval
AI is no longer confined to text-based retrieval! Multimodal RAG extends capabilities to process and integrate images, videos, and spatial data, enabling richer and more dynamic AI interactions.
4. Graph-Based RAG – Structured Knowledge Mastery
Instead of relying purely on unstructured document search, Graph RAG structures information into knowledge graphs. This allows for more meaningful contextual relationships, providing AI with a deeper understanding of how concepts interconnect.
5. Hybrid RAG – The Best of Multiple Search Methods
Hybrid RAG combines vector-based retrieval, keyword search, and other hybrid techniques to refine accuracy. This approach minimizes errors, making AI responses more reliable and contextually precise.
6. Agentic RAG – The Next Frontier
This cutting-edge model integrates autonomous AI agents that dynamically manage complex queries, routing them across multiple data sources. By acting as intelligent information routers, these AI agents deliver hyper-optimized and context-aware responses.
The Future of RAG: Key Trends Dominating 2025
AI-driven retrieval and generation are continuously evolving, with several emerging trends pushing the boundaries of efficiency, accuracy, and adaptability. Here’s what’s shaping the future of RAG:
1. Dynamic Retrieval Pipelines – Context-Aware Adaptation
RAG is shifting from static retrieval models to dynamic systems that adjust based on user queries, intent recognition, and real-time contextual data. This enables AI to provide more precise, on-demand responses.
2. Self-Optimizing RAG – Smarter Learning Mechanisms
Future RAG models are learning from past interactions, improving their retrieval processes with every iteration. These self-optimizing AI systems continuously refine their knowledge selection, drastically reducing hallucinations and misinformation.
3. Multi-Agent RAG – Collaborative Intelligence
The era of isolated RAG models is over! In 2025, multiple specialized RAG systems will work together in AI ecosystems, ensuring responses are cross-verified and highly refined. Each RAG instance will specialize in different domains, allowing for more diverse and accurate results.
4. Knowledge Graph Fusion – Merging Symbolic and Neural AI
Graph-based RAG systems are evolving to fuse structured and unstructured data sources. By integrating symbolic AI (knowledge graphs) with deep learning-based neural retrieval, AI systems can process information more comprehensively and accurately.
5. Multimodal RAG – AI That Understands the World in Multiple Dimensions
Text-only retrieval is quickly becoming obsolete. AI models are now integrating video, audio, spatial, and even real-world sensory data to create more immersive and interactive search experiences. This shift towards multimodal reasoning is opening new doors for AI in fields like robotics, augmented reality, and autonomous systems.
Conclusion: RAG Is No Longer Just Retrieval + Generation
Retrieval-Augmented Generation is no longer a one-size-fits-all approach. The landscape is evolving rapidly, with specialized models catering to different industries, data types, and knowledge systems. Whether it’s self-learning AI, multimodal integration, or agentic intelligence, the future of RAG is a dynamic ecosystem that orchestrates information in ways never seen before.
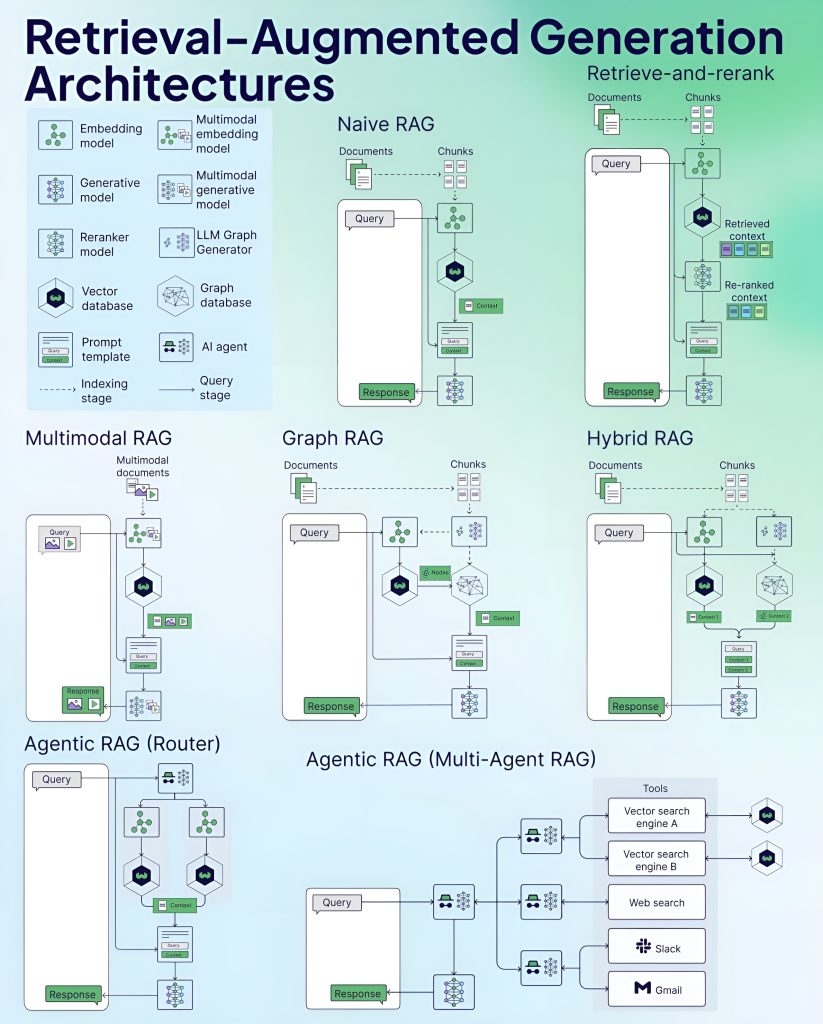
What’s your take on the future of RAG? Will Agentic RAG dominate AI-driven knowledge retrieval? Drop your thoughts in the comments below! Don’t forget to like, share, and subscribe for more cutting-edge AI insights. The future of AI is here, and you don’t want to miss it!
#AI #MachineLearning #ArtificialIntelligence #TechTrends #RAGTechnology